I am creating an neural style transfer AI artist in this tutorial, to be able to create a new image from a combination of two images. Neural style transfer (NST) is a machine learning algorithm that adopts a visual style to another image or video. NST is used to create artificial artwork by combining a content image and a style reference image.
Neural Style Transfer was introduced in 2015 by Leon A. Gatys, Alexander S. Ecker and Matthias Bethge, the algorithm was published in A Neural Algorithm of Artistic Style. The authors used a convolutional neural network (CNN) with a VGG19 architecture, the model was pretrained on the ImageNet dataset.
Dataset and Libraries
I am using a pretrained VGG19 model with weights from ImageNet in this tutorial. The dataset consists of a photograph and a style reference image, images is shown below. I chosed to use 256×256 images in order to get fast training time. I am using the following libraries: os, time, argparse, numpy, keras and scipy
.
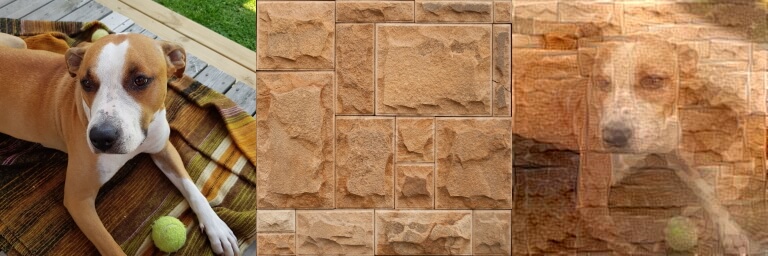
Training
I chosed to set the content image weight to 30 % and the style reference image weight to 70 %, the target size of the combined image is 256 rows times 256 columns. I have been running the code in 200 iterations (10, 10, 80, 100) and the output image is shown to the right in the image above. The result from a run is shown below the code.
# Import libraries
import os
import time
import argparse
import numpy as np
import keras
import keras.preprocessing
import scipy.optimize
# Evaluator class that makes it possible to compute loss and gradients in one pass
class Evaluator(object):
# Initialize the class
def __init__(self, rows:int, cols:int, outputs:[]):
self.loss_value = None
self.grads_values = None
self.rows = rows
self.cols = cols
self.outputs = outputs
# Calculate loss
def loss(self, x):
loss_value, grad_values = eval_loss_and_grads(x, self.rows, self.cols, self.outputs)
self.loss_value = loss_value
self.grad_values = grad_values
return self.loss_value
# Calculate gradients
def grads(self, x):
grad_values = np.copy(self.grad_values)
self.loss_value = None
self.grad_values = None
return grad_values
# The gram matrix of an image tensor (feature-wise outer product)
def gram_matrix(x):
# Turn a nD tensor into a 2D tensor with same 0th dimension
if keras.backend.image_data_format() == 'channels_first':
features = keras.backend.batch_flatten(x)
else:
features = keras.backend.batch_flatten(keras.backend.permute_dimensions(x, (2, 0, 1)))
# Return gram matrix
return keras.backend.dot(features, keras.backend.transpose(features))
# Preprocess an image
def preprocess_image(path:str, rows:int, cols:int):
# Load the image
x = keras.preprocessing.image.load_img(path, target_size=(rows, cols))
# Convert to array
x = keras.preprocessing.image.img_to_array(x)
x = np.expand_dims(x, axis=0)
# Proprocess with a VGG19 model
x = keras.applications.vgg19.preprocess_input(x)
# Return the image
return x
# Deprocess an image
def deprocess_image(x, rows:int, cols:int):
# Reshape image
if keras.backend.image_data_format() == 'channels_first':
x = x.reshape((3, rows, cols))
x = x.transpose((1, 2, 0))
else:
x = x.reshape((rows, cols, 3))
# Remove zero-center by mean pixel
x[:, :, 0] += 103.939
x[:, :, 1] += 116.779
x[:, :, 2] += 123.68
# Convert BGR to RGB
x = x[:, :, ::-1]
x = np.clip(x, 0, 255).astype('uint8')
# Return the image
return x
# Calculate style loss
def style_loss(style, combination, rows:int, cols:int):
# Calculate input values
S = gram_matrix(style)
C = gram_matrix(combination)
channels = 3
size = rows * cols
# Return style loss
return keras.backend.sum(keras.backend.square(S - C)) / (4.0 * (channels ** 2) * (size ** 2))
# Calculate content loss
def content_loss(base, combination):
return keras.backend.sum(keras.backend.square(combination - base))
# Calculate total variation loss
def total_variation_loss(x, rows:int, cols:int):
# Element-wize squaring
if keras.backend.image_data_format() == 'channels_first':
a = keras.backend.square(x[:, :, :rows - 1, :cols - 1] - x[:, :, 1:, :cols - 1])
b = keras.backend.square(x[:, :, :rows - 1, :cols - 1] - x[:, :, :rows - 1, 1:])
else:
a = keras.backend.square(x[:, :rows - 1, :cols - 1, :] - x[:, 1:, :cols - 1, :])
b = keras.backend.square(x[:, :rows - 1, :cols - 1, :] - x[:, :rows - 1, 1:, :])
# Return the total loss
return keras.backend.sum(keras.backend.pow(a + b, 1.25))
# Evaluate loss and grads
def eval_loss_and_grads(x, rows:int, cols:int, outputs:[]):
# Reshape image
if keras.backend.image_data_format() == 'channels_first':
x = x.reshape((1, 3, rows, cols))
else:
x = x.reshape((1, rows, cols, 3))
# Get loss value
outs = outputs([x])
loss_value = outs[0]
# Get gradient values
if len(outs[1:]) == 1:
grad_values = outs[1].flatten().astype('float64')
else:
grad_values = np.array(outs[1:]).flatten().astype('float64')
# Return loss and gradient values
return loss_value, grad_values
# The main entry point for this module
def main():
# Variables
base_image_path = 'C:\\DATA\\Python-data\\neural-style-transfer\\images\\giana256x256.jpg'
style_image_path = 'C:\\DATA\\Python-data\\neural-style-transfer\\styles\\abstract-asymmetry-brown-cement.jpg'
output_image_path = 'C:\\DATA\\Python-data\\neural-style-transfer\\images\\giana-cement-style.jpg'
total_variation_weight = 1.0
style_weight = 0.7
content_weight = 0.3
iterations = 100
# Get base image size and set target size
width, height = keras.preprocessing.image.load_img(base_image_path).size
rows = 256
cols = int(width * rows / height)
# Preprocess images
base_image = keras.backend.variable(preprocess_image(base_image_path, rows, cols))
style_image = keras.backend.variable(preprocess_image(style_image_path, rows, cols))
output_image = None
# The output_image will contain our generated image
if keras.backend.image_data_format() == 'channels_first':
output_image = keras.backend.placeholder((1, 3, rows, cols))
else:
output_image = keras.backend.placeholder((1, rows, cols, 3))
# Combine 3 images into a single Keras tensor
input_tensor = keras.backend.concatenate([base_image, style_image, output_image], axis=0)
# Build the VGG19 network with 3 images as input
model = keras.applications.vgg19.VGG19(input_tensor=input_tensor, weights='imagenet', include_top=False)
print('VGG19-model has been loaded!')
# Get the symbolic outputs of each layer (we gave them unique names)
outputs_dict = dict([(layer.name, layer.output) for layer in model.layers])
# Combine loss functions into a single scalar
loss = keras.backend.variable(0.0)
layer_features = outputs_dict['block5_conv2']
base_image_features = layer_features[0, :, :, :]
combination_features = layer_features[2, :, :, :]
loss = loss + content_weight * content_loss(base_image_features, combination_features)
feature_layers = ['block1_conv1', 'block2_conv1', 'block3_conv1', 'block4_conv1', 'block5_conv1']
# Loop layers and calculate loss
for layer_name in feature_layers:
layer_features = outputs_dict[layer_name]
style_reference_features = layer_features[1, :, :, :]
combination_features = layer_features[2, :, :, :]
sl = style_loss(style_reference_features, combination_features, rows, cols)
loss = loss + (style_weight / len(feature_layers)) * sl
# Get total loss
loss = loss + total_variation_weight * total_variation_loss(output_image, rows, cols)
# Get the gradients of the generated image
grads = keras.backend.gradients(loss, output_image)
# Get outputs
outputs = [loss]
if isinstance(grads, (list, tuple)):
outputs += grads
else:
outputs.append(grads)
# Create an evaluator
evaluator = Evaluator(rows, cols, keras.backend.function([output_image], outputs))
# Get input image
if(os.path.isfile(output_image_path) == True):
x = preprocess_image(output_image_path, rows, cols)
else:
x = preprocess_image(base_image_path, rows, cols)
# Loop for a predefined number of iterations
for i in range(iterations):
# Print start
print('Start of iteration', i + 1)
# Get starting time
start_time = time.time()
# Run scipy-based optimization (L-BFGS)
x, min_val, info = scipy.optimize.fmin_l_bfgs_b(evaluator.loss, x.flatten(), fprime=evaluator.grads, maxfun=20)
# Print loss value
print('Current loss value: ', min_val)
# Deprocess image
img = deprocess_image(x.copy(), rows, cols)
# Save generated image
keras.preprocessing.image.save_img(output_image_path, img)
# Print iteration done
print('Iteration {0} completed in {1} seconds'.format(i + 1, round(time.time() - start_time, 2)))
# Tell python to run main method
if __name__ == '__main__': main()
VGG19-model has been loaded!
Start of iteration 1
Current loss value: 297102530.0
Iteration 1 completed in 31.57 seconds
Start of iteration 2
Current loss value: 282029000.0
Iteration 2 completed in 30.82 seconds
Start of iteration 3
Current loss value: 278050500.0
Iteration 3 completed in 30.69 seconds
Start of iteration 4
Current loss value: 276365820.0
Iteration 4 completed in 30.83 seconds
Start of iteration 5
Current loss value: 275439400.0
Iteration 5 completed in 31.58 seconds
Start of iteration 6
Current loss value: 274867260.0
Iteration 6 completed in 31.47 seconds
Start of iteration 7
Current loss value: 274493700.0
Iteration 7 completed in 31.94 seconds
Start of iteration 8
Current loss value: 274209700.0
Iteration 8 completed in 32.48 seconds
Start of iteration 9
Current loss value: 273964220.0
Iteration 9 completed in 32.9 seconds
Start of iteration 10
Current loss value: 273742050.0
Iteration 10 completed in 32.52 seconds